Navigating Bias in AI
by Saile Villegas, Co-Founder / CEO
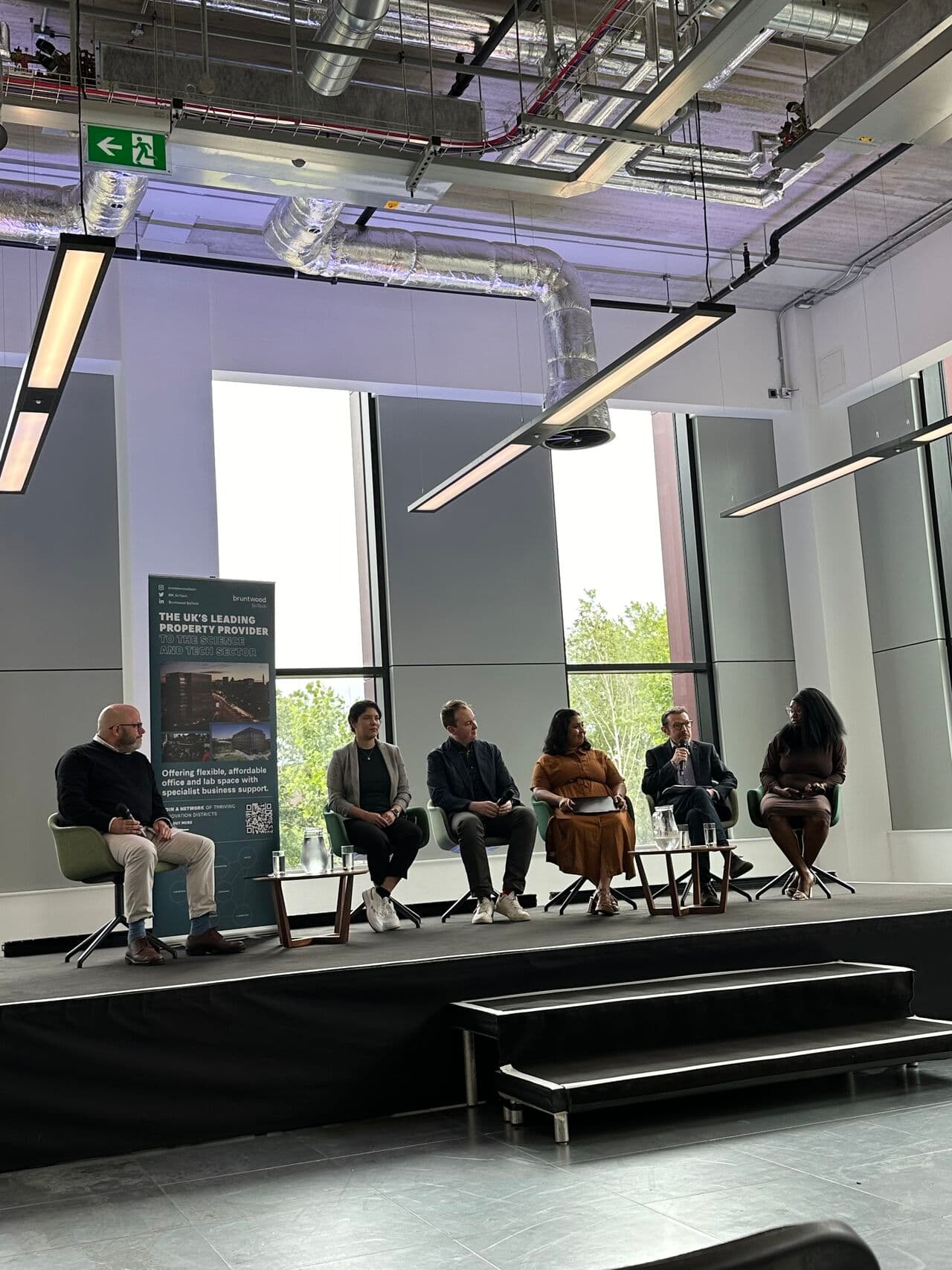
The history of Artificial Intelligence (AI) dates back many decades. More recently, however, society has begun to embrace it as an everyday essential - a trend accelerated by the rise of generative AI tools such as the large language model (LLM) ChatGPT.
Despite a more gradual growth in its early development, AI technology is now progressing at pace. And this presents a wealth of opportunities and challenges for those that use it.
As part of Seeai's commitment to operating responsibly, we like to lead and learn from discussions that explore the impact of AI in more detail. Recently, our co-founder Saile Villegas joined a panel at Bruntwood SciTech in Birmingham, to discuss the everchanging outcomes of AI, with a particular focus on the issue of bias. In this article, we'll share our top takeaways from this informative event.
AI has both positive and negative outcomes
The session started with an introduction from Toju Duke, Responsible AI Advocate, Author and Founder of Diverse AI. During her insightful keynote speech, she covered a brief history of the technology and highlighted its ability to do both good and harm in the world. Examples of which are outlined below.
AI used for good:
- Health and hunger, security and justice, economic empowerment, education, environment, public and social sectors, information verification, crisis response...
AI's challenges:
- Social inequities, privacy violations, energy consumption, disinformation, human rights violations, copyright violations psychological safety, democracy...
Guidelines are needed
Given the possible issues and opportunities AI presents, it's important that we have the right rules and regulations in place to guide its development. And, as creators, educators and users of AI technology, it's our responsibility to put those guidelines in place.
As an example, Toju shared her thoughts on what a responsible AI framework could look like.
- AI principles: putting governing principles in place for AI and working to protect them.
- Data: ensuring we use data sets that are built and reviewed correctly.
- Fairness: checking to see if the results of AI are fair to all groups.
- Safety: testing vigorously to ensure the results of AI are safe for all users.
- Explainability: ensuring transparency and accountability are seen as standard practices.
- Human-in-the-loop: ensuring humans are included in the building of the process.
- Privacy and robustness: ensuring the models and systems we create are robust enough to withstand attacks and prevent data leaks.
- AI ethics: being aware of energy consumption, annotator wellbeing.
Navigating Bias in AI
Following this session, a panel of speakers took to the stage to discuss the specific topic of bias in AI. Expert speakers included:
- Panel Chair: Professor Jack Grieve PhD, University of Birmingham
- Saile Villegas, Seeai
- Chris Alderson, AND Digital
- Oyinkansola Adebayo, Niyo Group
- Peter Ross, Lokulus
- Abinaya Sowriraghavan, Aston University
What is bias in AI?
Bias in AI refers to the systematic and unfair prejudice in the outcomes of AI algorithms, often stemming from the data used for training or designing the model. These biases can manifest in various forms, such as racial, gender, or socio-economic discrimination, and can lead to unequal treatment or representation in automated decision-making systems.
Examples of bias manifesting itself in AI include:
-
Geographical bias Many of the conversational products powered by generative AI that are being utilised by the public right now (Such as ChatGPT or Claude) are developed in the Western world. This means they tend to embody Western values and concepts. They may also perform better when conversed with in English, given that they were predominately trained using English language text. This can put less well-represented geographies and languages at a disadvantage.
-
Gender bias In other research, when asked to produce advertising scripts for a range of products, a large language model (LLM) was found to be more likely to generate female-gendered words when describing products such as furniture polish and washing machines whereas male-gendered words were strongest for lawnmowers and electric drills.
What causes this bias?
AI models are trained using human data and, as humans, we all show bias to some degree. From our location to our education, many factors can cause us to develop certain skews in our perspective and AI will inherit this before serving it back to us as content or 'information'.
How can we navigate this bias?
As an industry, it's our job to stop the cycle of bias and put systems in place that test both the input and outputs of AI. Here we list 10 of the many ways you can approach this.
1. Have principles in place
In the earlier days of generative AI, the BBC set out three guiding principles before undertaking any development work in this area. Firstly, everything the organisation does has to be in the interest of the general public. Secondly, it must work in a very open and transparent way. And finally, the use of AI must supplement human work and not replace jobs. Having your own set of principles in place will help you keep your core values and vision intact no matter where the technology takes you.
2. Get the foundations right
For those of us developing AI products and solutions, it's vital that we build responsibly. This means starting with robust, inclusive data before deploying any technology. What's more, we need to ensure that the annotators who label the content and data we use in our models are paid and treated fairly.
3. Prioritise testing
Many people are calling for more regulation in the field of AI. Even the big companies are deploying technology with major issues in place, due to the lack or strength of testing. However, if we want to develop products that are ethical and effective, testing and transparency are key.
AI is constantly evolving, what behaves today may not behave tomorrow and the problems we train out of our models can be replaced by new issues incredibly quickly. We must be vigilant and ensure we never become complacent in testing the technology. Where possible, we also need to share best practices for testing within the industry to help counteract the speed of AI's evolution.
4. Crunch the numbers
AI Bias can be very subtle. In fact, it's often undetectable if you see results in isolation. As such, it's worth investing in statistical analysis to see where certain patterns or peaks emerge in your product, rather than spot testing, so that you can address any issues that may arise.
AI bias can be subtle. In fact, it's often undetectable if you see results in isolation. As such, it's worth investing in statistical analysis to see where certain patterns or peaks emerge in your product, rather than relying on spot testing alone, so that you can address any issues that may arise. For a more thorough evaluation, consider implementing fairness tests. These tests, such as demographic parity or equal opportunity metrics, help assess whether your AI treats different groups equally. They can detect subtle biases across various demographic categories that might not be apparent from surface-level observations. By incorporating these tests into your analysis, you can better ensure the fairness and equity of your AI system.
5. Keep humans in the loop
As organisations creating AI products, we must stay alert and monitor for bias at every stage, from data to deployment. With a diverse team of humans overseeing your processes and product development, you're more likely to catch any issues.
As an AI user, it's equally important to involve humans when utilising the technology and not just blindly accept the decisions or outputs AI can make for you. We can't just automate tasks within AI and forget about them as it will lead to faults and failings.
6. Educate users
There is a divide forming between people who understand the products we're building and the impact they could have and those who don't. As a result, some people are utilising AI without realising the harm it could do them, either now or in the future. If we want to continue developing and deploying emerging technologies at speed, it's our responsibility to upskill and educate all users, including ourselves, about the pros and cons they present. Only then will we ensure everyone is in a fair position and the technology is used correctly.
7. Educate the models
We can address bias in AI models through various methods, including supervised learning with carefully curated datasets, rigorous testing, and ongoing monitoring. However, bias can also emerge in unexpected ways, so it's crucial to implement comprehensive strategies for bias detection and mitigation throughout the AI development and deployment process.
8. Implement local models
By deploying open-source models on-premises, companies gain greater control over their AI systems, allowing organisations to utilise curated training datasets and have more control of their inputs and outputs. This approach minimises risks associated with data transmission over the internet and enhances privacy. Local implementations give organisations full control over model versions and updates, enabling them to fine-tune models to their specific needs, providing a robust solution for organisations prioritising data privacy and bias mitigation.
9. Ask questions
It's important that we see AI technology as a tool in its trial and error phase, and not a universal solution for all issues. We need to take the time to question its use, results and the decisions it makes for us. If we don't exercise our critical thinking and rely too heavily on AI outputs, we could end up experiencing complacency. As a result, issues like bias will go unchecked more often.
10. Use the resources available
Keeping up to speed is key in navigating the world of artificial intelligence. To do this, you can make the most of free AI training or pay to attend courses from organisations such as the British Computer Society. There are great free online courses available in platforms like DeepLearning.AI, offering Andrew NG's Generative AI for everyone that covers how generative AI works and how to use it in your life and at work. Also worth making the most of educational resources such as the AI ethics and government in practice paper from the Alan Turing Institute. Tools such as Google's What If are also helpful when testing a model.
In summary, AI has the ability to benefit a lot of people but it's advancing at speed. By taking the time to question, test and improve our work we can ensure its potential for good is optimised and any negative outcomes, such as bias, are minimised. If you have any further questions about AI and how you can make the most of it in your organisation, please do get in touch.